COM_MIC_DEPARTMENT_TITLE
講題
利用模型及類神經網路進行磁振影像輔助之正子斷層資訊優化——以失智症群體為例
Using Models and Neural Networks
for MR-Assisted PET Data Optimization in Dementia
講者
程子翔 Kevin T. Chen, Ph.D.
Center for Advanced Functional Neuroimaging
Radiological Sciences Laboratory
Stanford University
時間
12/22(五)13:30
地點
校總區生物科技館R101 (長興街81號)
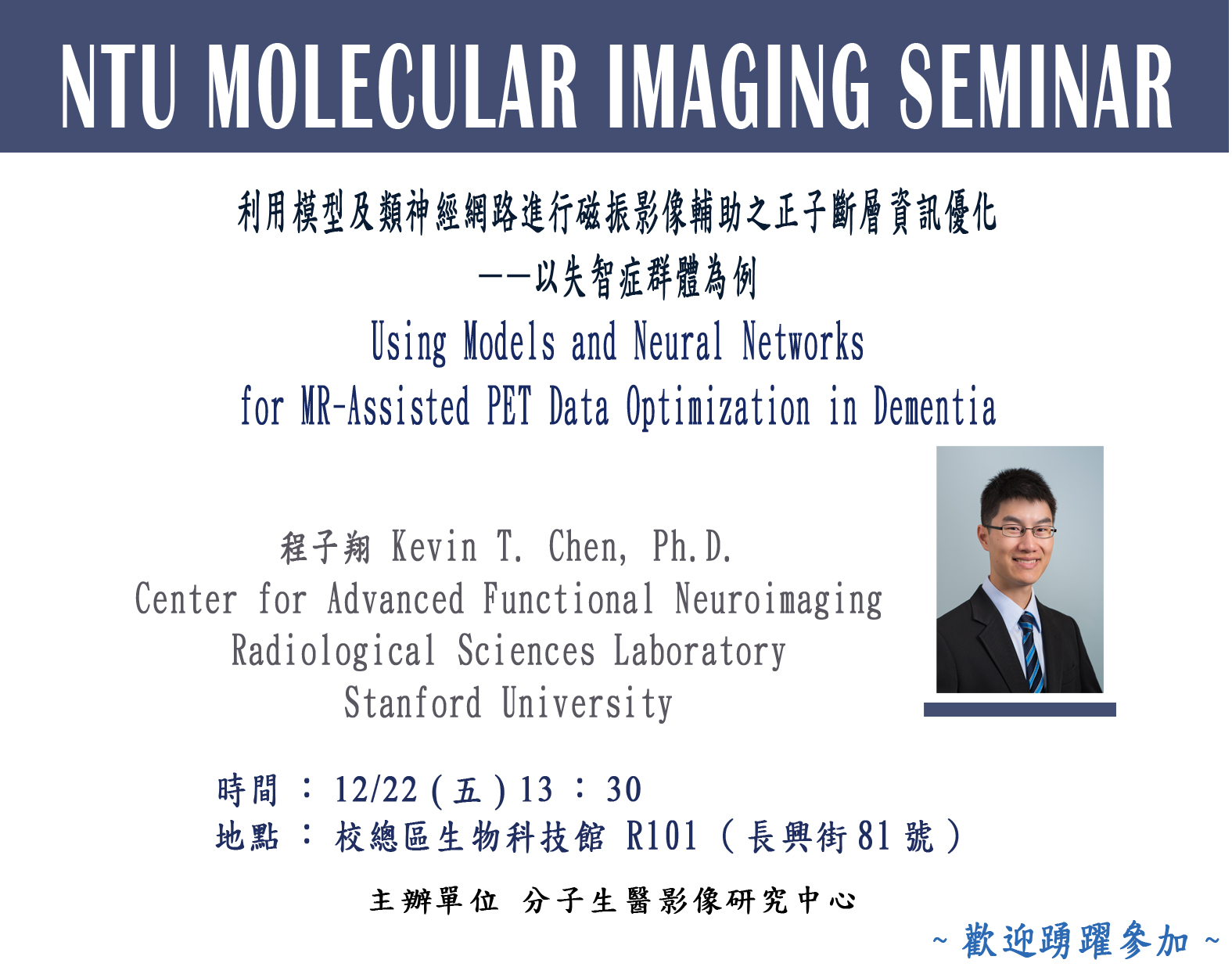
COM_MIC_TEXT_TITLE
Abstract
Recent advances have allowed the hardware integration of positron emission tomography (PET) and magnetic resonance imaging (MRI). Though PET has many advantages, including quantitatively assessing in vivo biological processes, its accuracy is confounded by several factors while exposing the subject to ionizing radiation. For example, attenuation correction is required to account for the interactions of the annihilation photons in the subject; motion correction is needed to minimize image degradation due to subject movements; partial volume effects correction is required due to the relatively limited spatial resolution. However, the spatiotemporally-correlated MRI data can be utilized to improve the PET scanner performance. Out of numerous potential applications, this talk will focus on dementia as MRI and PET are widely used and provide complementary information in the assessment of these patients. Equally important, dementia is a great test situation for these methodological developments because the confounding factors mentioned above are especially pronounced in this patient population. In the first part of this talk, a unified protocol to address these limitations will be introduced. Specifically, methods to derive head attenuation maps from the morphological MR images and the utilization of temporally-correlated MR data for PET motion compensation and spatially-correlated MR data for anatomy-aided reconstruction will be discussed. After applying these tools to data acquired in dementia patients, the PET image quality improved substantially and the group variability in PET measurements was reduced. Finally, with deep neural networks, we propose to use multiple MR images and a noisy, ultra-low-dose amyloid PET image to synthesize a high-quality PET image resembling that acquired with typical injected dose. This technique can potentially increase the utility of hybrid amyloid PET/MR imaging in clinical diagnoses and longitudinal studies.